Anyscale Launches Ray Train and Ray Data Dashboards for Enhanced Observability
Joerg Hiller May 20, 2025 03:51
Anyscale introduces Ray Train and Ray Data Dashboards, offering new features for improved observability and performance optimization in distributed AI model training and data pipelines.
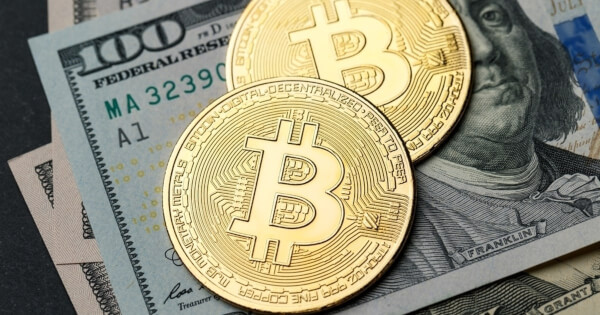
Anyscale has unveiled its new Ray Train and Ray Data Dashboards, designed to simplify debugging and enhance performance tuning for distributed AI model training and data processing. According to Anyscale, these dashboards provide a unified interface to monitor and optimize machine learning workflows.
Enhanced Observability with Ray Train Dashboard
The Ray Train Dashboard offers four key observability features: training progress visualization, error attribution, comprehensive logs and metrics, and profiling tools. These tools allow users to drill down into worker-level behavior, making it easier to identify performance bottlenecks. For instance, integrated tools like dynolog
enable Torch training runs to be profiled efficiently.
This dashboard addresses the complexity of monitoring distributed training jobs, which often requires manually correlating scattered logs and metrics. By providing a unified interface, the Ray Train Dashboard simplifies this process, allowing users to access logs and metrics from both the Train Controller and Worker processes from a single platform.
Ray Data Dashboard for Data Pipeline Optimization
The Ray Data Dashboard introduces Tree and Directed Acyclic Graph (DAG) views, along with operation-level metrics and dataset-aware log aggregations. These features help machine learning engineers quickly identify bottlenecks and optimize data pipelines, which are fundamental to AI applications.
With the new dashboard, teams can easily visualize their data pipeline's structure, monitor progress, and pinpoint inefficiencies. This functionality is crucial for debugging and optimizing large-scale data processing workloads, which are often complex and resource-intensive.
Future Enhancements and Integration Plans
Both dashboards are set to evolve with future enhancements, including automated issue detection and integration with experiment tracking platforms like Weights & Biases and MLflow. These improvements aim to provide even deeper insights and more robust tools for managing distributed AI systems.
Anyscale's new dashboards are available on their platform, offering powerful tools for AI practitioners to build, optimize, and scale their systems with increased efficiency. These advancements mark a significant step in simplifying the management of distributed AI workloads, enabling users to focus more on innovation and less on troubleshooting and performance issues.
Image source: Shutterstock